Coarse Grained Force Field Optimization using Machine Learning Methods
Date: Nov. 9, 2023, 2:11 p.m.
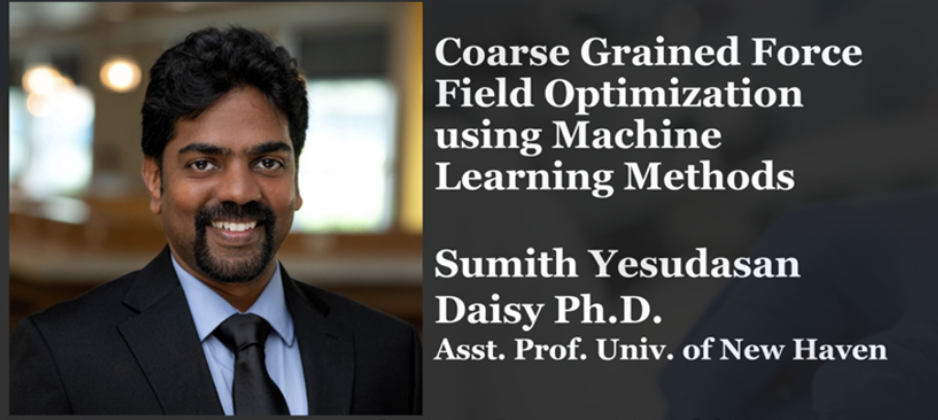
Seminar Details: The development of accurate and efficient force fields is essential for the simulation of molecular processes in computational biophysics and materials science. Coarse-grained (CG) models offer a promising approach by reducing the complexity of molecular systems, allowing for the investigation of larger systems and longer timescales. However, the simplification inherent in CG models necessitates careful parameterization to ensure that essential physical properties are retained. Traditional force field optimization relies heavily on iterative, manual adjustments based on intuition and comparison with experimental or high-level theoretical data, which can be time-consuming and may not capture the multidimensional nature of the parameter space. This presentation will explore the integration of machine learning (ML) methods into the optimization of CG force fields. We will discuss how ML algorithms can be employed to systematically improve force field parameters by learning from a diverse set of training data, including structural, thermodynamic, and dynamic properties of molecules. The focus will be on the application of Genetic Algorithm, to predict the quality of force field parameters and guide the optimization process.
Details
Dr. Yesudasan is an Assistant Professor of Mechanical Engineering at the University of New Haven, with a rich background that encompasses a decade of expertise split between academia and industry. He holds a PhD in Mechanical and Aerospace Engineering from Syracuse University. His research is multifaceted, focusing on the development of advanced force fields for water, the intricacies of radiative heat transfer, and the complex modeling of blood clots. With five years of prior professorship and an equal span in the industrial sector, Dr. Yesudasan brings a balanced perspective to his teaching and a practical edge to his innovative research endeavors.